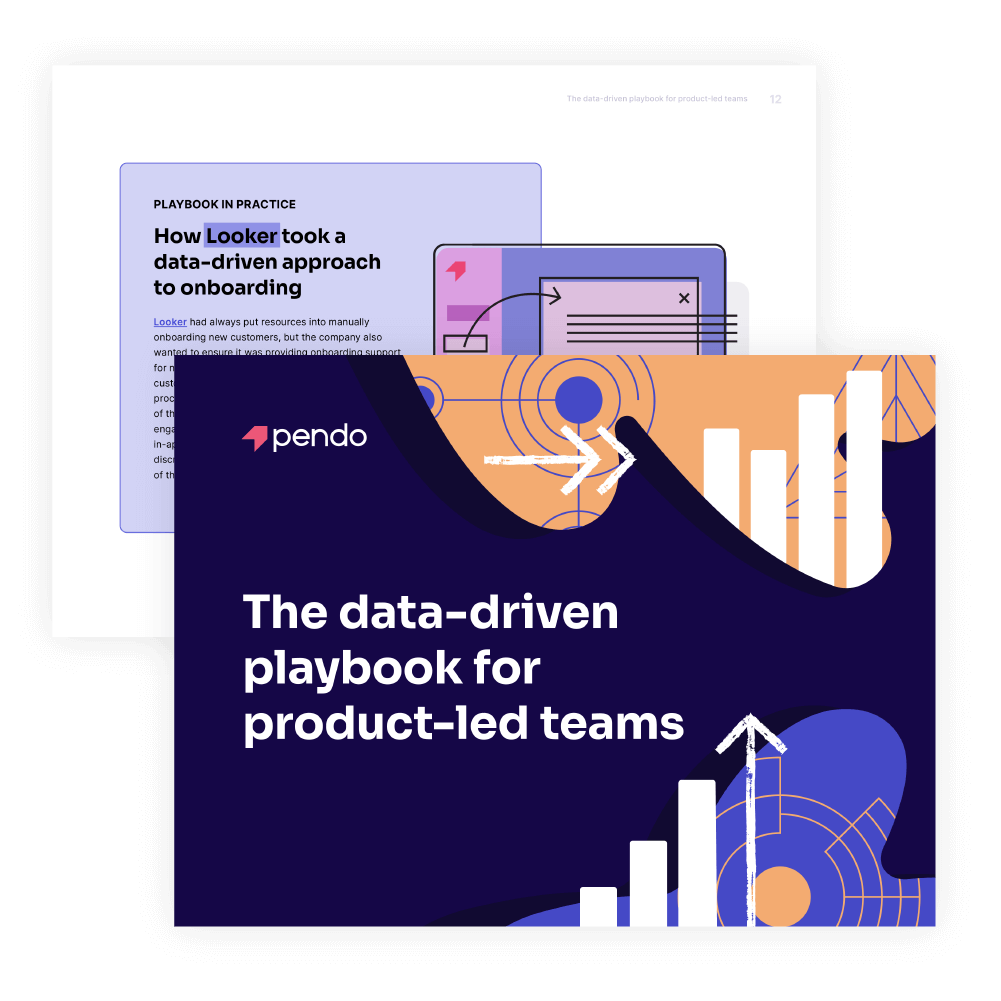
Inhaltsverzeichnis
In a rush?
Download the PDF for later
Introduction
What’s a product-led company to do with all that data?
They say you can’t improve what you don’t measure. At product-led organizations, there’s no shortage of measurement going on. Teams across the business—from product, engineering, and IT to customer success, sales, and marketing—are constantly looking to product usage data and customer feedback to inform their work.
Rather than accessing data on one-off occasions or searching for a data point that confirms an existing opinion, product-led companies leverage data throughout the entire product development lifecycle and customer journey. Here’s what that might mean for different teams:
- A product manager (PM) examines user behavior to identify friction points in a particular workflow and determine what changes need to be made to the user interface (UI). They then create an in-app survey to ask users about their experience with the product and how it could be improved.
- A customer success manager (CSM) looks at their account’s product usage leading up to a quarterly business review, so they can come into the meeting with actionable recommendations and go-forward plans.
- An IT leader monitors employees’ usage with a particular tool in order to determine if the company is making the most of its software investment, or if they should remove it from the tech stack.
- A customer marketer utilizes product usage data to identify power users who would be good candidates to speak at an event, join a community, or leave a review on a third party site. They immediately follow up with a tailored in-app guide to make the request.
- A sales rep leverages usage data from the company’s freemium product to identify users who are the most engaged and likely to be interested in the full platform. From there, they create in-app guides that speak to the value of paid features and direct users to where they can go to upgrade.
As exemplified above, being data-driven means taking action on the data you collect. Beyond product analytics, this requires specific tools (or ideally, a single tool) that allow you to communicate with and collect feedback from users and customers inside your product. This then fosters a continuous loop of measuring, testing, collecting feedback, and iterating—creating a virtuous cycle for product-led organizations.
Throughout the rest of this playbook, we’ve curated tactics and best practices that you can use to take action on all the valuable data you’re collecting—or could be collecting. It’s organized into five sections, each with different “plays” that ladder up to the broader theme.
Let’s get started.
Product and feature launch
By nature, launches are celebratory occasions. But at product-led companies, they’re less of an endpoint and more of a stop along the iteration journey. Rather than working up to one big release, your product team is likely dripping out capabilities over multiple stages and exposing new features to additional users over time. For example, they might do an internal release, limited beta, open beta, and finally open it up to general availability.
This iterative approach to releasing products and features means there’s even more opportunity to leverage data every step of the way. Here are some tactics to add to your toolkit:
Use data to target launch announcements
While teams use multiple channels (e.g. email, blog posts, social media) to let customers know about a new product or feature, product-led companies know that delivering these announcements in-app is one of the most effective methods. And data can make these communications even more impactful.
For in-app launch announcements, make sure you’re targeting messages to users who will find the new feature most valuable or to whom the new functionality is most relevant. Your product likely serves multiple types of users (e.g. with different roles, maturity, and levels of technical proficiency), and very few features will be relevant to all users.
Use data from a product analytics tool to understand users’ behavior, then build segments for in-app guides based on what you learn. For example, if a new feature is meant to complement an existing feature, start by targeting users who currently use the feature to let them know about this new functionality and how it will improve their workflows.
Iterate based on in-app guide engagement
Once your announcement guides are live in the product, you can take a data-driven approach to improving them. Measure engagement with in-app announcements to understand how your launch campaigns are performing, and if guides are successfully grabbing users’ attention. There are three metrics we recommend tracking:
- Guide views
- Time in guide
- Guide step completion (if your guide has multiple steps)
If a guide has low engagement, it might be worth moving it to a different location in the product to see if users react better when it’s introduced at a different point in their workflow. It’s also useful to experiment with different copy and imagery, or even update the segmentation to see if a different set of users engages with the guide more. On the other hand, use guides with high engagement as a learning opportunity: What do you believe is making them successful, and how can you apply these learnings to the rest of your in-app announcements?
Track product usage post-launch
Rather than creating in-app launch announcements and hoping for the best, it’s crucial to track product usage after a launch to measure the success of your efforts—and identify how you can improve.
Start by looking at how many customers are using the new feature (we recommend first looking one week after the launch) and if it’s contributing to increased engagement with the product. By looking at usage patterns, you’ll have a better sense of what customers might still need from the product. For example, what users do before and after accessing the feature or how long they spend with it can indicate where there is friction or a missing capability. If you see low adoption rates initially, you might also need to create additional in-app guides to remind users about what’s new and even provide a link that’ll take them directly to the feature or product area.
Collect feedback to add context to quantitative inputs
While teams are busy collecting and analyzing quantitative data throughout every phase of a release, you can’t forget to pair it with qualitative feedback from your users themselves. This feedback is instrumental in informing how you iterate and improve new functionality, especially as the competitive landscape, market trends, and users’ needs evolve.
With each release phase, make sure you’re collecting feedback to understand what users like, what’s not working, or how the product or feature could be improved. This not only helps the product team know where to make improvements, but helps teams like marketing or customer success adjust launch communications based on what they know users find valuable about the new product or feature. Similar to any launch announcements, it’s best to collect this feedback directly within the product using in-app surveys, polls, or prompts that let users provide written feedback.
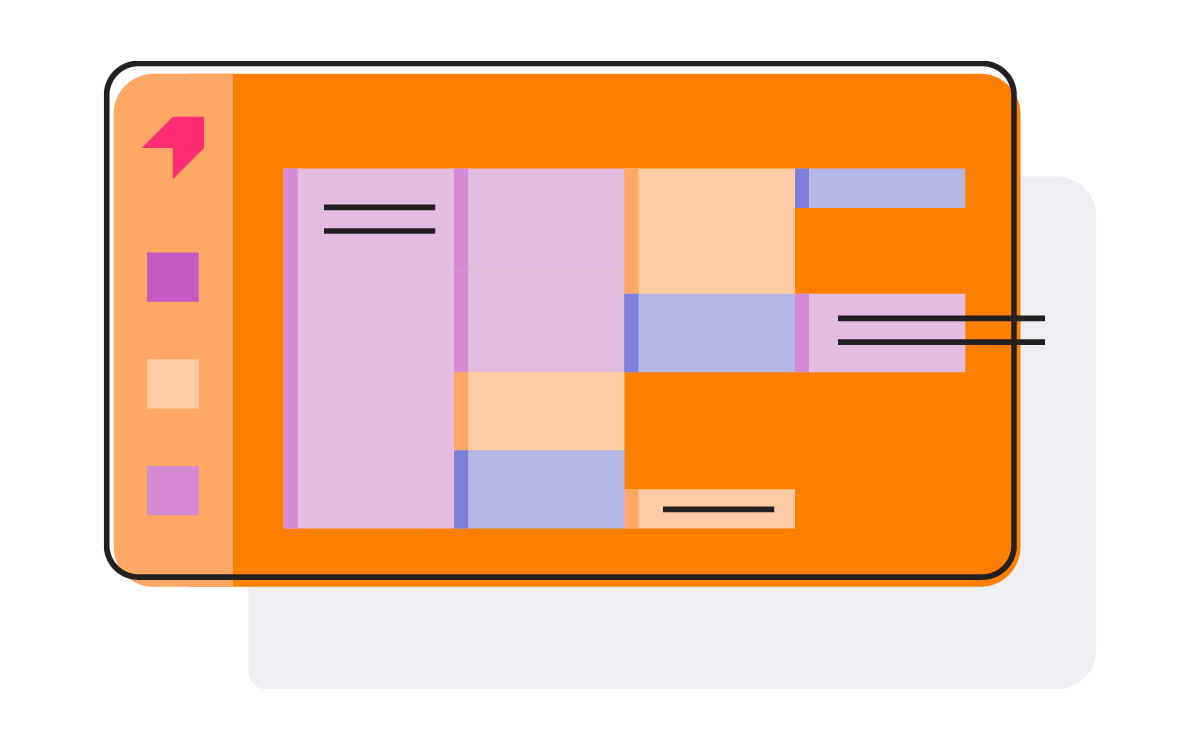
PLAYBOOK IN PRACTICE
How Global Payments used data to iterate post-launch
After releasing a new feature, Global Payments needed to know how users were engaging with it to ensure the feature was delivering on its intended value and solving the right pain points. They looked at user journeys and saw that after using the new Payment Request feature, 50% of users were then searching for the transaction to make sure it went through. This highlighted what was missing: the ability to send a notification when a payment is completed. With this insight, the Global Payments product team had the information they needed to iterate quickly and improve this functionality for their customers.
Onboarding
Instead of thinking about onboarding as a moment in time, product-led companies view onboarding as a critical part of a user’s journey: when they become proficient and start finding value in your product. To make onboarding as impactful as possible, it’s first important to bring it inside the product itself. With product-led onboarding, companies can accelerate time to value for their users by providing contextual information as they use and navigate the product.
When you start to view onboarding as a discrete experience and deliver it in-app, plenty of opportunities arise to leverage data to inform and optimize this work.
Choose the right features to highlight
As you build out in-app onboarding, one of the first key steps is choosing which features and product areas to introduce to new users. It’s best to think about quality over quantity—you shouldn’t show new users all of your product’s features, but rather those that are needed to accomplish the most important tasks and workflows.
Use product analytics data to see which features your existing users are engaging with frequently and getting the most value from. There are multiple ways to determine your “most popular” features, but one common approach is to see which features generate 80% of the click volume in your application. You can also use product data to better understand which actions in the product correlate with outcomes like positive customer sentiment, retention, expansion, or trial conversion, and ensure onboarding flows direct new users to (and explain the value of) those actions. For example, if you’re able to segment product usage data by sentiment, take a look at the features your Net Promoter Score (NPS) Promoters use the most and how this list compares to your list of most popular features.
Personalize based on what you know about users
Just like the product experience as a whole, onboarding should be customized to users’ needs—which all begins with data. Whether your product serves users with different job titles, permission levels, or otherwise, in-app onboarding is most effective when it caters to an individual’s specific use case. This includes both your onboarding content (e.g. which features you highlight and how you talk about them) and how it is delivered (e.g. would certain types of users prefer to first explore the app on their own, then view a guided walkthrough later on?).
You can leverage what you already know about your users to craft different onboarding flows. Here are some data points that might inform how you segment in-app onboarding:
- Berufsbezeichnung
- Berechtigungsstufe innerhalb des Produkts
- Vertikale Branche
- Free trial start/end date
- Time spent in the application
- Features used
Segmenting in this way not only ensures your onboarding content will be as relevant as possible, but also helps avoid cluttering the UI with unnecessary information that can be distracting or frustrating for new users.
Measure onboarding effectiveness
After all the work you put into creating an in-app onboarding experience that’s engaging, customized, and value-driven, data can help you track its effectiveness and understand where there is room for improvement. Think about measuring onboarding in three ways: engagement with onboarding content, impact on product usage, and resulting business outcomes.
First, it’s helpful to know how users are engaging with your onboarding content. Are they getting through all of the walkthroughs? Where is there drop off? Similar to measuring engagement with in-app launch announcements, there are three guide metrics you should focus on: guide views, guide step completion, and time in guide. This data will help indicate if you’re reaching your intended audience and whether or not new users are finding value in your onboarding content.
Second, you’ll want to know if onboarding is positively impacting how customers use the product post-onboarding. Track usage for the features included in your onboarding flows—ideally, feature usage will have increased or remained relatively steady over time. You can also use funnels to measure how users move through the steps that were introduced during onboarding.
Finally, you can tie onboarding to business metrics like free trial or freemium conversion rates, support costs, NPS, and retention. For example, do customers who engage with onboarding content generate fewer support tickets? Does onboarding completion correlate with long-term product engagement and/or a lower likelihood to churn? Examining data in these ways will help you start to see the larger business impact your onboarding program is making.
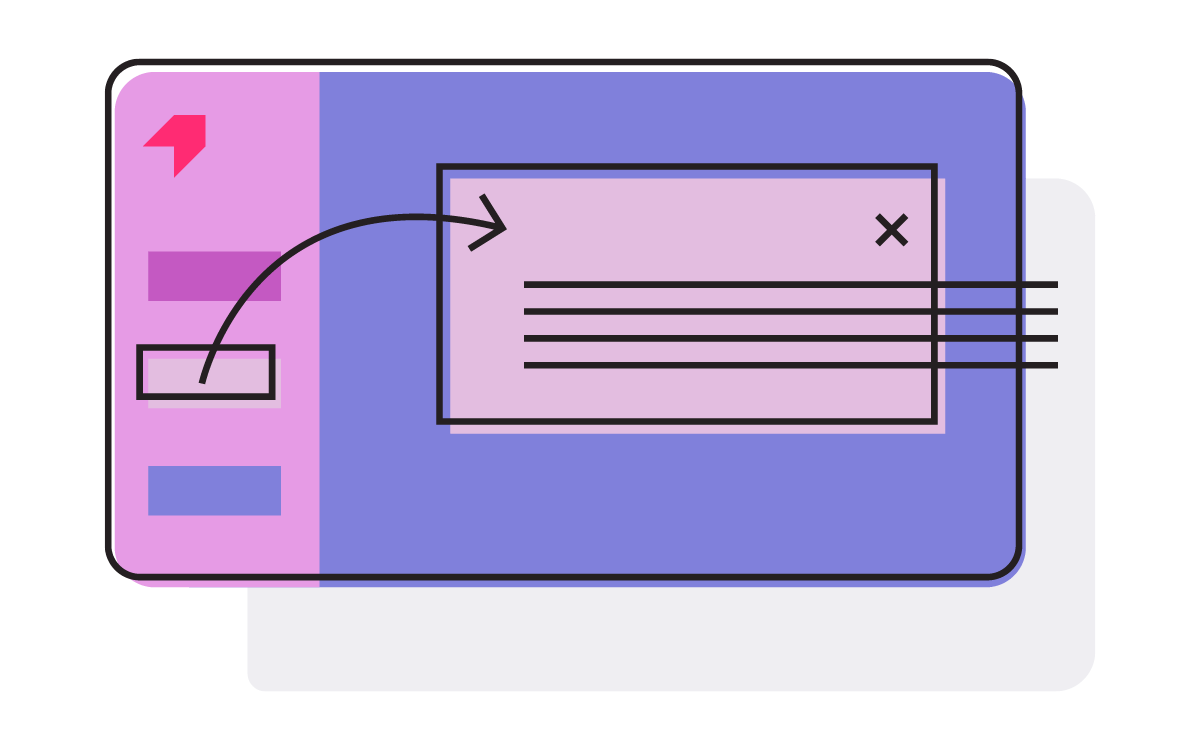
PLAYBOOK IN PRACTICE
How Looker took a data-driven approach to onboarding
Looker had always put resources into manually onboarding new customers, but the company also wanted to ensure it was providing onboarding support for new users within existing customer accounts. The customer success team used data to identify key processes that would best demonstrate the power of the product to new users, while also keeping them engaged and wanting to learn more. They then built in-app guides that broke these processes down into discrete steps, helping new users realize the full value of the powerful, complex tool.
Akzeptanz
In the past, product teams often measured success based on the number of features shipped. But product-led companies know that adoption is a much more useful metric—and is critical for teams beyond just the product organization.
Well-adopted products help users discover value quickly, keep those users coming back, and encourage habitual usage as the product becomes part of their regular routine. Adoption is about more than simply increasing usage of all or any of your product’s features. It’s about driving adoption of the right features—those that create value for your users, contribute to positive sentiment, and lead to customer outcomes like retention and expansion. This first and foremost hinges on the availability of data, and the ability to take action on it.
Get a baseline measure of adoption
When it comes to improving adoption, the first step is putting the mechanisms in place to properly measure it. Start by getting a baseline measure of both feature and product adoption, which will allow you to spot any initial trends and compare against these metrics in the future.
Product adoption is best expressed over time by the total number of monthly active users (MAU), weekly active users (WAU), or daily active users (DAU). You can also measure it as a rate relative to new user signups for a given period of time. A common way to measure feature adoption is by the percentage of features that generate 80% of your product’s total click volume. With this initial list of top features, there are three additional dimensions to consider:
- Breadth of adoption: How widely has a feature been adopted across your user base or targeted user segment?
- Time to adopt: When learning about a feature, do users immediately try it out or do they wait several days or weeks before picking it up?
- Duration of adoption: How long do users continue to use a feature after learning about it?
Once you have a sense of both product and feature adoption, you’ll be able to use this data to inform how you launch new offerings, educate users in-app, and improve short- and long-term adoption rates.
Examine user journeys so you know where to intervene
In addition to tracking product and feature adoption, you’ll want to form hypotheses around what typical and ideal user journeys look like in your product. To make sense of your adoption data, it’s helpful to approach it with specific questions in mind. Here are a few to consider:
- Which features are most important for delivering customer value?
- What are the key workflows or actions that a user should complete?
- How do those workflows evolve throughout a customer’s lifecycle?
- Do our most used features align with expectations?
Compare your predictions with actual user behaviors, leveraging user path analyses to see the journey to or from a certain feature or page in your application. You can also compare user journeys for different segments of your user base, for example users who have and have not used a key feature. From there, you’ll be able to uncover points of friction that may be preventing optimal adoption of the product or feature.
Drive positive workflows and behaviors in-app
Once you have insight into how users are adopting your product and its features, it’s equally important to ensure they are set up to utilize the product to its fullest potential. Product, marketing, and customer success teams each have a role to play here—they all have a vested interest in customers fully adopting and seeing value in the product.
Use in-app messages to encourage positive workflows and behaviors while users are actively engaged with the product. In areas where you see users getting stuck, you can add contextual tooltips or full in-app walkthroughs to help clear up any confusion and guide users through the workflow. For features with low adoption, you can take a similar approach with an added emphasis on discoverability. Create in-app guides that live in product areas with high engagement, and educate users on other features that will help them complete tasks faster or more effectively. Segmentation is particularly useful here, as you can target these in-app messages to groups of users who will find the feature most valuable.
Use data to identify products or features to sunset
It’s not uncommon for product teams to largely focus on effectively launching and driving adoption of products and features. But sometimes, the most strategic move is to actually remove a feature from the UI or retire a product entirely. As you dig into adoption data, take note of any pages or features that have low or no activity over 90+ days—these may be good candidates for a potential sunset.
While low usage can prompt a decision to sunset a feature, it’s important to first consider the context. Low usage levels might be a sign of discoverability and usability problems, not necessarily that users no longer find the functionality valuable. Try to determine the reasons for low usage before flagging a feature to be removed, and even consider asking users directly if (or why) they find the particular feature valuable.
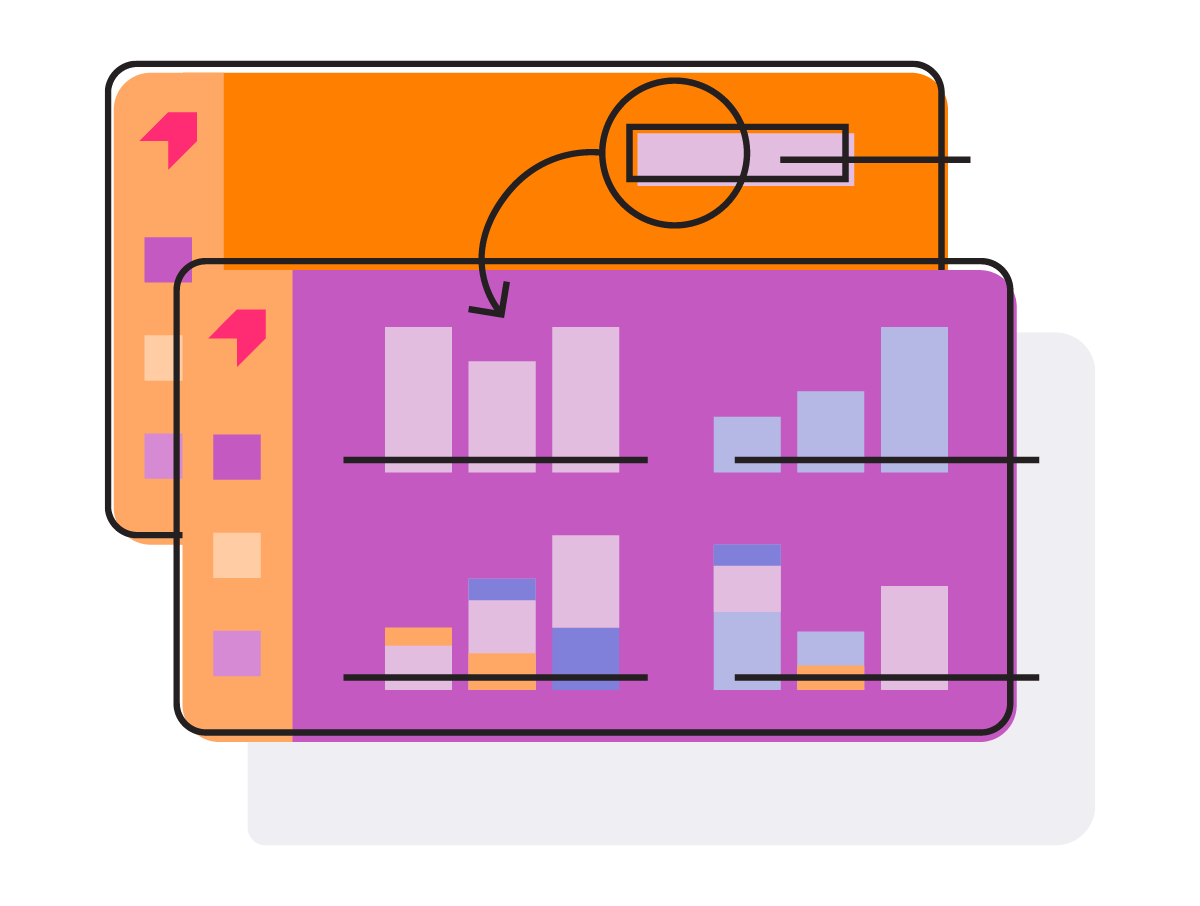
PLAYBOOK IN PRACTICE
How MineralTree put feature adoption data into action
After launching an exciting new feature, initial adoption data signaled to the MineralTree team that the feature’s location was making it difficult for users to find it. With limited engineering resources and lagging adoption rates, MineralTree took action on this data and used an in-app guide to “reposition” the feature on their dashboard page—helping users easily locate the new functionality from the page they visit most often. Since launching the guide, MineralTree has seen a 75-100% increase in traffic to the new feature.
Feedback and roadmapping
Product managers are no stranger to feedback coming at them from all directions—from customers and internal teams alike. Rather than simply finding a way to organize all of this feedback, product-led companies put processes in place to centralize feedback and make it actionable for everyone across the organization.
This helps companies take a data-informed approach to prioritization and product development. Rather than relying on gut instinct, product leaders can prioritize key initiatives and features based on direct user feedback, and teams like sales, customer success, and marketing can use feedback to inform their own work, too. And while qualitative feedback is data in and of itself, the real power comes from combining feedback with quantitative inputs like product usage data to ensure teams continually focus their efforts on the right things.
Target your in-app feedback collection
Product-led companies use the product as the ultimate communication channel, including to collect feedback from their users. In addition to creating an “always-on” way for customers to provide feedback whenever they’d like, teams should use in-app guides and surveys to proactively ask users for their input—and data can help make this outreach even more impactful.
Leverage product usage data to target in-app feedback requests to users who can provide the most valuable input. Depending on which area of your product you’re seeking feedback on, product usage data can help you determine your ideal respondents. For example, if you’re looking for feedback on how to improve a certain feature, you’ll want to solicit feedback from users who have actually engaged with the feature. Or if you want to collect feedback about your onboarding experience, it’s best to target users who are new to the product and most recently went through onboarding.
Segment feedback data for deeper insights
When it comes to analyzing feedback data, one of the most powerful tools at a team’s disposal is segmentation. In addition to viewing customer feedback as a whole, separate it in order to learn what different types of customers are asking for. You can segment feedback data by things like company size, annual recurring revenue (ARR), role, industry, NPS response, assigned CSM, feature usage, or subscription type (if you have a free and paid version of your product).
The way you segment will largely depend on both your business’ overarching goals and if there are any specific initiatives you’re looking to launch or iterate on. For example, if your company is trying to move up market and target larger enterprise customers, you’ll want to look at feedback from these accounts specifically to see if there are any patterns. If your product team is looking for feedback after a recent feature launch, start by evaluating feedback from users who have engaged with the new feature.
Pair feedback with usage data to prioritize your roadmap
Engineering resources are always limited, forcing product teams to focus on maximizing customer value for every feature they deliver. The tricky part, though, is knowing which items on the roadmap will deliver the most value.
In general, understanding how much an existing feature or area of the product is used should inform whether or not to invest additional development resources. But that doesn’t mean features with low usage should be completely ignored—a valuable feature may be underused simply because users are getting tripped up during one part of the workflow.
Without a clear view into how users are really engaging with your product—like where they’re spending the most time or where they’re getting stuck—it’s difficult to know which feedback items and requests will have the greatest impact. By combining qualitative feedback with quantitative usage data, you’ll be able to understand the “why” behind observed behavior and better prioritize your roadmap.
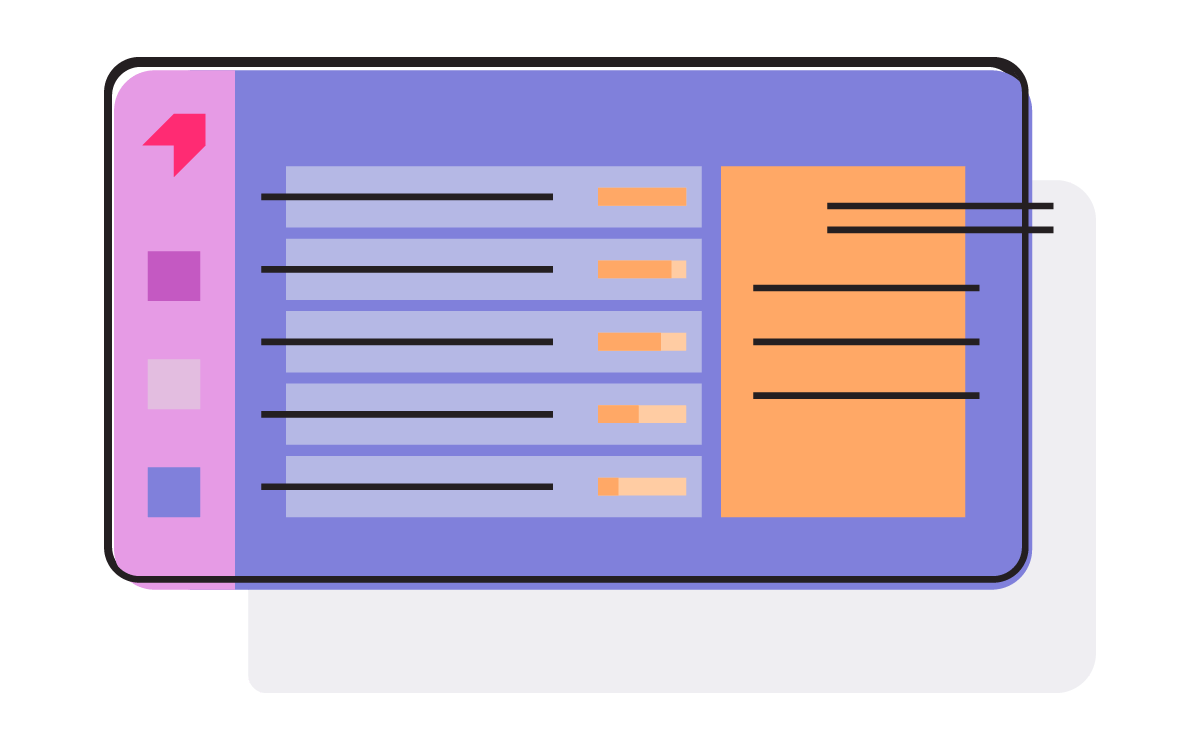
PLAYBOOK IN PRACTICE
How Filevine uses qualitative and quantitative data to make better decisions
In order to gather and act on customer feedback in a scalable, data-driven way, Filevine implemented a feedback management tool so they could better serve their highly engaged user base. Now, they’re able to combine qualitative feedback and quantitative usage data to prioritize product improvements and make decisions more quickly. And by having a centralized view of all feedback and requests, Filevine can easily see if a problem is affecting all of their customers or determine if users would benefit from a new feature—a process that took 40 to 50 hours in the past.
Wachstum
When companies are product led, they lean on the product to deliver the entire customer experience—including opportunities to drive growth. This helps increase efficiency by freeing up internal sales and marketing resources and creating a clear path to conversions directly within the product.
Free products (including free trials, freemium, and product tours) play an outsized role in driving product-led growth. They let people try out a product before purchasing it, and give users the chance to see the product’s value and experience how it can solve their problems. Just like a company’s paid product, teams can leverage data to more effectively build and manage their free offering.
Use data to set your freemium product’s usage threshold
Since freemium products give users access to part of a product for an unlimited amount of time, teams are tasked with deciding what to include in the freemium experience. The goal is to offer enough to demonstrate the product’s value, but still leave users wanting more.
When deciding which parts of the product freemium users should have access to, you can use data to inform your approach. Look at product usage data from your paid product to identify average usage patterns, then think about what a scaled down version of certain product areas could look like. For example, if your average customer creates five reports in your application per month, you can set the threshold at three reports and require free users to upgrade if they want to create any more. If you see that a certain feature is only really accessed by users with advanced skills, that might be a good feature to leave out of your freemium product.
Encourage upgrades at the right moments
One of the biggest benefits of free products is that they allow users to self-serve and upgrade or purchase right inside the product itself. The key is offering these upgrade options at the right moments in a user’s journey. Turn to product usage data to determine when and how to deliver in-app guides that notify users of upgrade options and inspire action.
If you’ve set a limit for a particular amount of usage in your freemium product (e.g. monthly active users), you can set up guides to automatically trigger when an account is close to reaching its limit. For free trials, dig into usage data to identify feature engagement that correlates with conversions to the paid product. From there, you can build in-app guides that steer trial users to those features in order to drive engagement and eventually, conversions. You can also create in-app guides that appear when users hover over or click on features that aren’t included in your freemium or free trial experience, letting them know that they need to upgrade to access this functionality and directing them to where they can go to do so.
Build a framework for product qualified leads
In addition to marketing and sales qualified leads (MQLs and SQLs), product-led organizations leverage product qualified leads (PQLs), which are leads that are scored based on engagement with the product. Create a framework for PQLs based on product usage data from both your free and paid products, and what you know about users who convert. For example, if there are certain features that users who convert to paying customers consistently access, those are valuable inputs for your PQL model.
Once you have a PQL model in place, your sales team can then use this data to target their outreach and identify which users are the most engaged, and therefore the most likely to be interested in the full paid platform. Since PQLs get to feel the product out for themselves, on their own terms, they’re warmer and more receptive to being sold to, compared to MQLs.
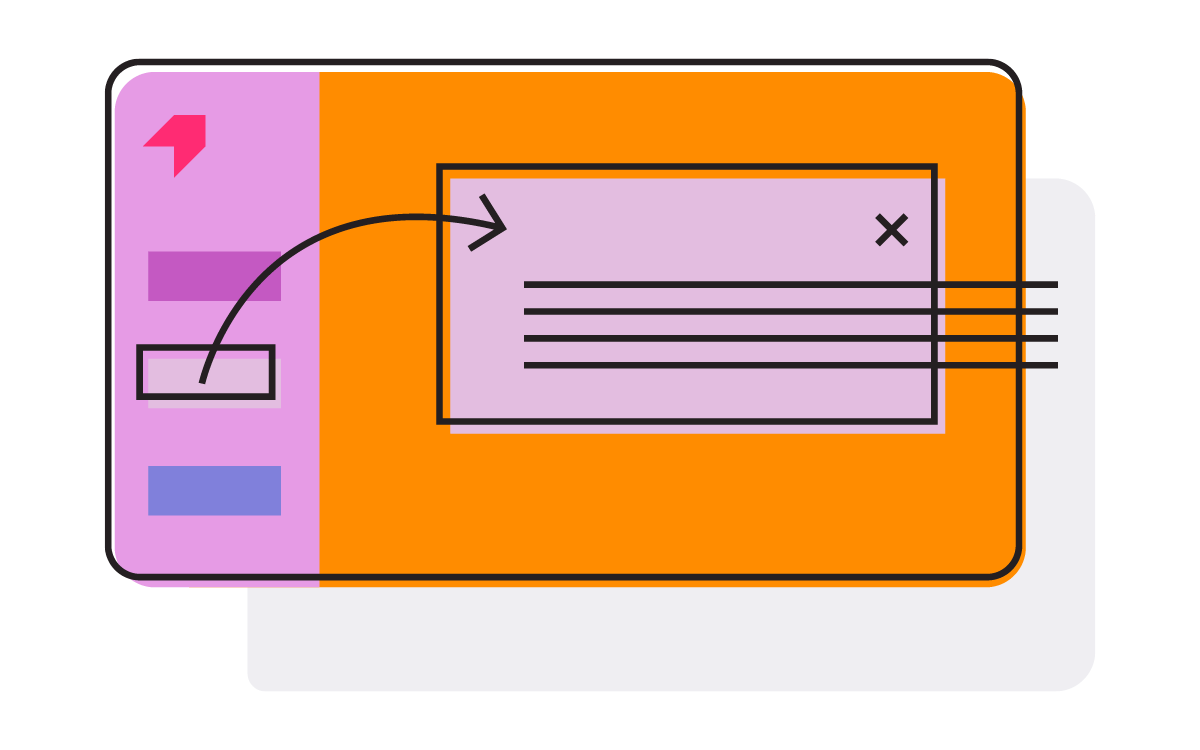
PLAYBOOK IN PRACTICE
How Citrix boosted trial conversions with personalized onboarding
Since users generate a free account for their ShareFile product for a variety of reasons, Citrix’s marketing team wanted to make these free trials more personalized to specific users’ preferences. Using paid search data, Citrix put together first-time use messaging based on why a user created their free account and leveraged in-app guides to walk users through the exact workflow they were searching for. After implementing these onboarding guides, Citrix saw a 60% increase in free trial conversions.